Date | 26 Aug 2024 |
Time | 5:00 pm - 6:00 pm (HKT) |
Venue | Lecture Theatre P2, Chong Yuet Ming Physics Building |
Speaker | Dr. Peichen ZHONG |
Institution | Baker Institute of Digital Materials for the Planet, University of California Berkeley |
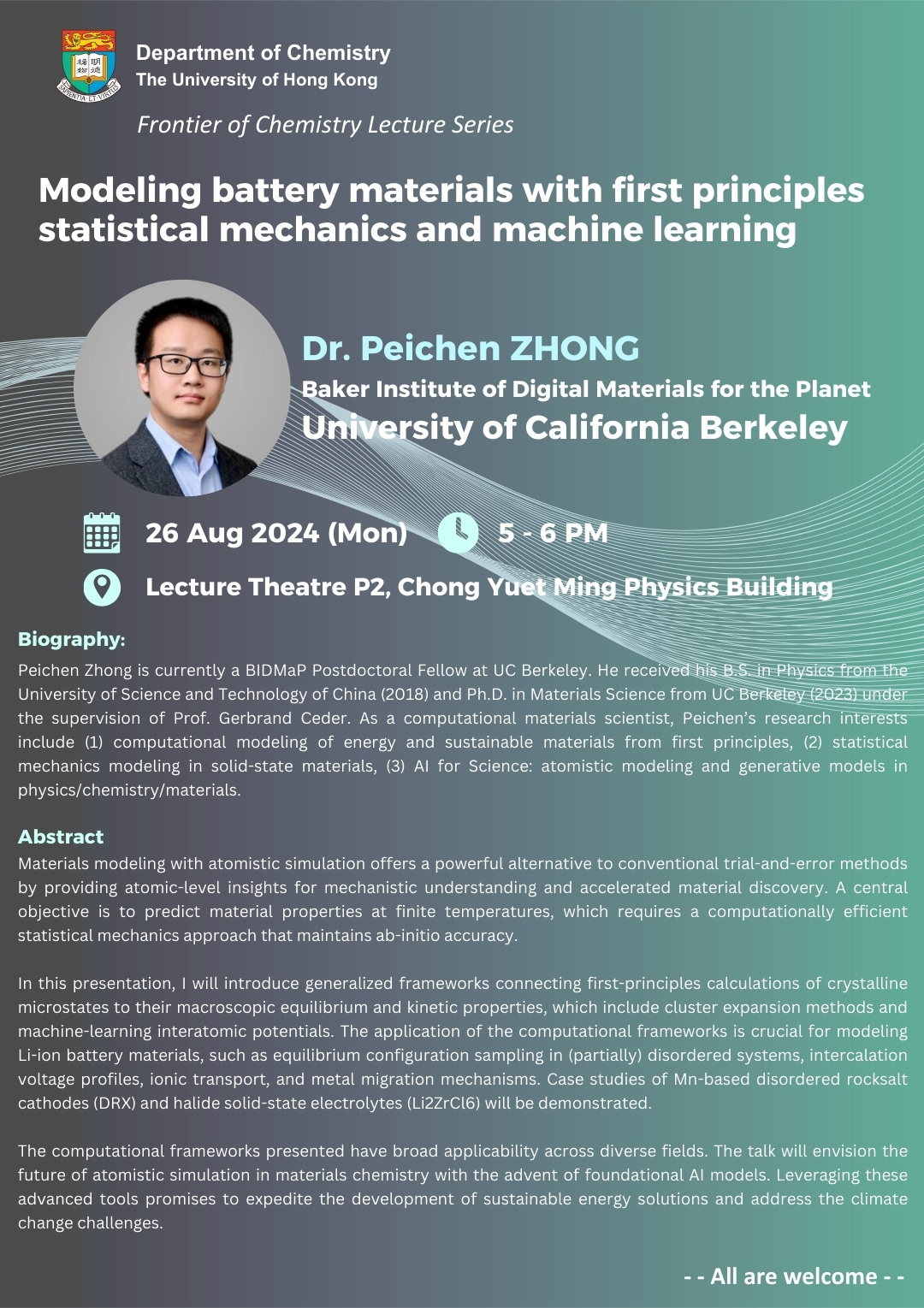
Peichen Zhong is currently a BIDMaP Postdoctoral Fellow at UC Berkeley. He received his B.S. in Physics from the University of Science and Technology of China (2018) and Ph.D. in Materials Science from UC Berkeley (2023) under the supervision of Prof. Gerbrand Ceder. As a computational materials scientist, Peichen’s research interests include (1) computational modeling of energy and sustainable materials from first principles, (2) statistical mechanics modeling in solid-state materials, (3) AI for Science: atomistic modeling and generative models in physics/chemistry/materials.
Materials modeling with atomistic simulation offers a powerful alternative to conventional trial-and-error methods by providing atomic-level insights for mechanistic understanding and accelerated material discovery. A central objective is to predict material properties at finite temperatures, which requires a computationally efficient statistical mechanics approach that maintains ab-initio accuracy.
In this presentation, I will introduce generalized frameworks connecting first-principles calculations of crystalline microstates to their macroscopic equilibrium and kinetic properties, which include cluster expansion methods and machine-learning interatomic potentials. The application of the computational frameworks is crucial for modeling Li-ion battery materials, such as equilibrium configuration sampling in (partially) disordered systems, intercalation voltage profiles, ionic transport, and metal migration mechanisms. Case studies of Mn-based disordered rocksalt cathodes (DRX) and halide solid-state electrolytes (Li2ZrCl6) will be demonstrated.
The computational frameworks presented have broad applicability across diverse fields. The talk will envision the future of atomistic simulation in materials chemistry with the advent of foundational AI models. Leveraging these advanced tools promises to expedite the development of sustainable energy solutions and address the climate change challenges.